Going Viral: How Social Media Is Making the Spread of the Coronavirus Worse
By Helen Lee Bouygues
April 2020
Introduction
The American public is central to stopping the spread of COVID-19 in the United States
To contain the disease and minimize its impact, people must distance themselves from others, wash their hands thoroughly, and avoid panic buying. They also need to stay home if they’re sick, cough into their elbows, and avoid touching their faces. As the tragic situation in Italy — where roughly more than 12,000 have died thus far — attests, the consequences of not taking precautions can be fatal for thousands.
Still, people have not been doing enough. In New York City, large groups recently gathered in parks. Farther south, college students crowded the beaches in Florida for typical spring-break activities. A number of Californians, told to stay home altogether, chose leisurely weekends at the beach over safety.
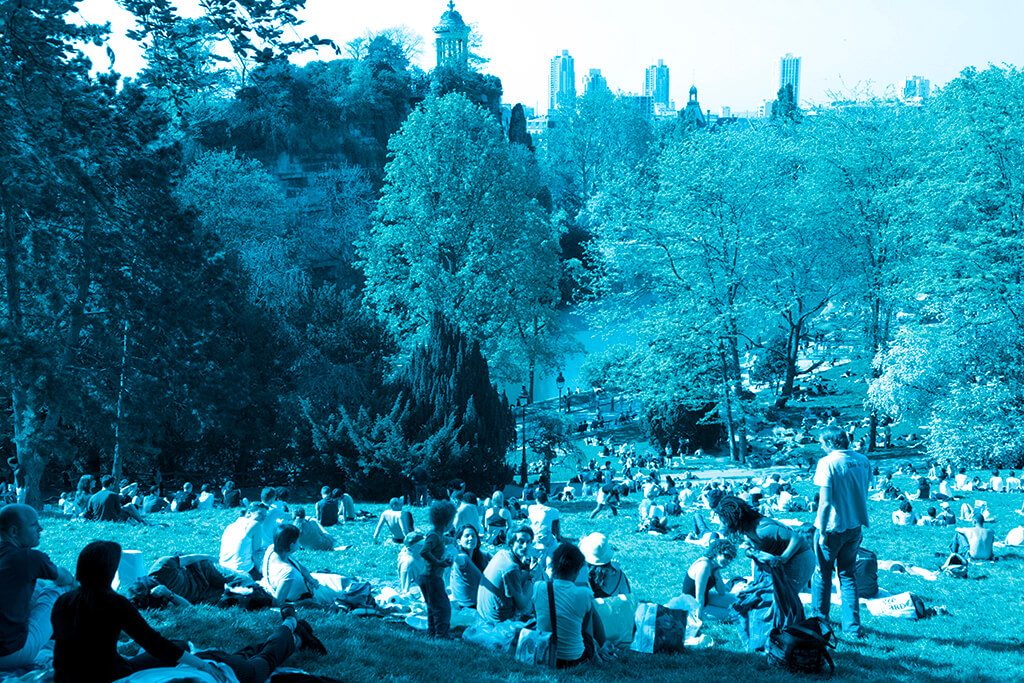
Many Americans have taken action, to be sure. In many places, restaurants, malls and schools have closed; people are practicing social distancing; and many cities have resorted to “shelter in place,” requiring residents to stay at home unless performing essential activities.
Still, containment of the disease ultimately relies on individual actions, and people need access to reliable factual information to make good decisions. To that end, the Reboot Foundation recently ran a number of analyses. First, it conducted a survey of the public on its knowledge of COVID-19 as well as its social media use. Second, the foundation scoured social media, tracking individual posts related to the virus. There were three main findings from our research:
Almost a third of the public believes in COVID-19 myths. There is a lot of misinformation about the coronavirus, and many Americans have a weak or ill-founded understanding of the virus. According to our representative survey of more than 1,000 people of various ages across the country, 29 percent, or almost a third, were misinformed on at least one aspect of the virus — and, in many cases, more.
The Reboot survey asked questions based on reliable information regarding COVID-19 — including from the Centers for Disease Control and Prevention (CDC) and the World Health Organization (WHO).
Unfortunately, an alarming number of those surveyed answered questions about COVID-19 incorrectly. For instance, 26 percent of respondents believed that COVID-19 will likely die off in the spring, and another 10 percent thought regularly rinsing their nose with saline will help prevent the virus. Another 12 percent believed that COVID-19 was created by people.
Many members of the public also did not believe that the disease would have a big impact on them or their friends and family, and roughly 20 percent believed that the coronavirus was not a serious issue, while only 18 percent thought that it was “extremely serious.”
Such myths existed even though people argued that they knew a lot about the illness, and there was a large metacognitive gap between what people thought they knew and what they actually knew.
For instance, more than 55 percent of respondents claimed that they were “very informed” or “extremely informed” about COVID-19. Another 42 percent said that they were “somewhat informed.” Only about 3 percent felt “not very informed” or “not at all informed.” In other words, people believed strongly that they knew the facts about the illness, but it turns out that, in many cases, their “facts” were wrong.
Given the current pandemic, such erroneous beliefs and deep misinformation among the public have consequences, and it will likely lead to a worsening of the crisis. For instance, if someone believes that the illness will die off in the warm weather, they will likely not engage in social distancing. Similarly, if someone believes that COVID-19 is not a serious illness, they might not engage in enough hand-washing.
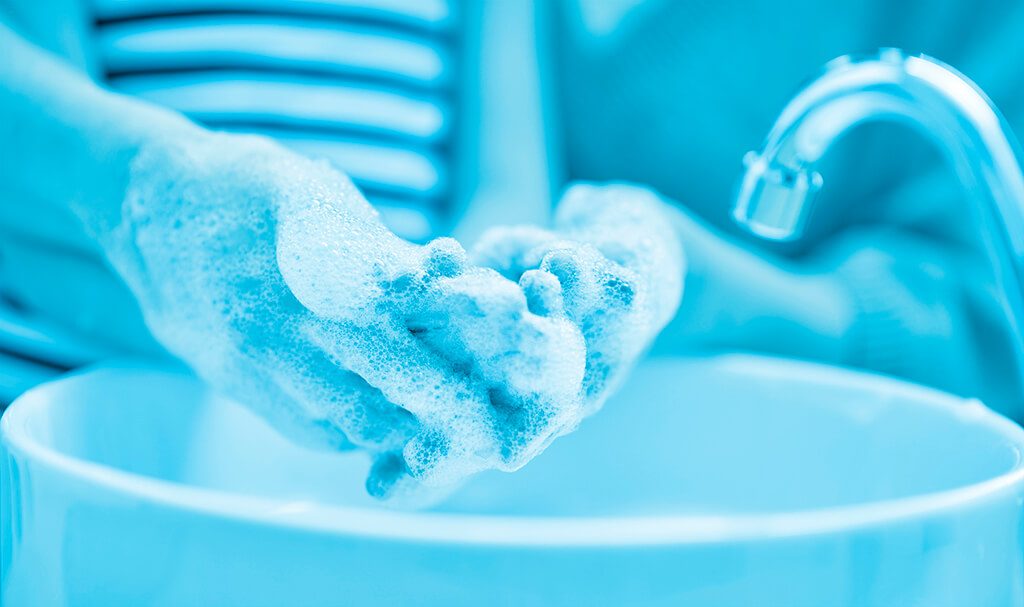
Social media use appears to drive misinformation around COVID-19. The more time people spend on social media, the more they believe in COVID-19 myths. This pattern was clear in the survey. An increase in social media use correlated with an increase in people being misinformed about the virus.
For example, 22 percent of those checking social media once a week harbored at least one wrong belief about the virus. By contrast, for those checking social media hourly or more frequently, that number jumped to 36 percent, or a difference of 14 percentage points.
Given the data, it’s not clear that social media use caused this change to occur. The data show correlations, not causation. But the analysis also showed that beliefs in myths about the virus were linked with the participants’ primary reliance on news sources. For example, people who relied on the CDC website for COVID-19 information answered more than 80 percent of the questions correctly. In contrast, heavy social media users only got 75 percent of the questions right.
Using regression analysis, we find that the effect of social media on a belief in COVID-19 myths is highly robust even when controlling for covariates such as age, education, and political ideology. What’s more, heavy social media users were far more likely to be misinformed over key facts. For instance, heavy social media users were significantly more likely to believe the virus was created by humans, and more likely to believe that items from China could contain the virus.
Part of the issue is that social media posts on the virus contain a lot of misinformation. One study released this month focused on Twitter and COVID-19 misinformation. That analysis showed that 25 percent of virus-related tweets contained information that was simply wrong. Another 17 percent of tweets disseminated information that could not be verified, according to the study.
The Reboot Foundation funded a different study showing similar results about fake health news on social media. In that study, which was released as a pre-print, researchers looked at all health-related posts on a single Facebook group — one of the most popular in Europe — and found that 28 percent of all posts related to health were inaccurate.
Another issue is that coronavirus information is being politicized. The results from the Reboot survey show, for example, that those who consider themselves conservative were more likely to believe in myths about the virus. They were also less likely to believe it would be serious if they were infected with COVID-19.
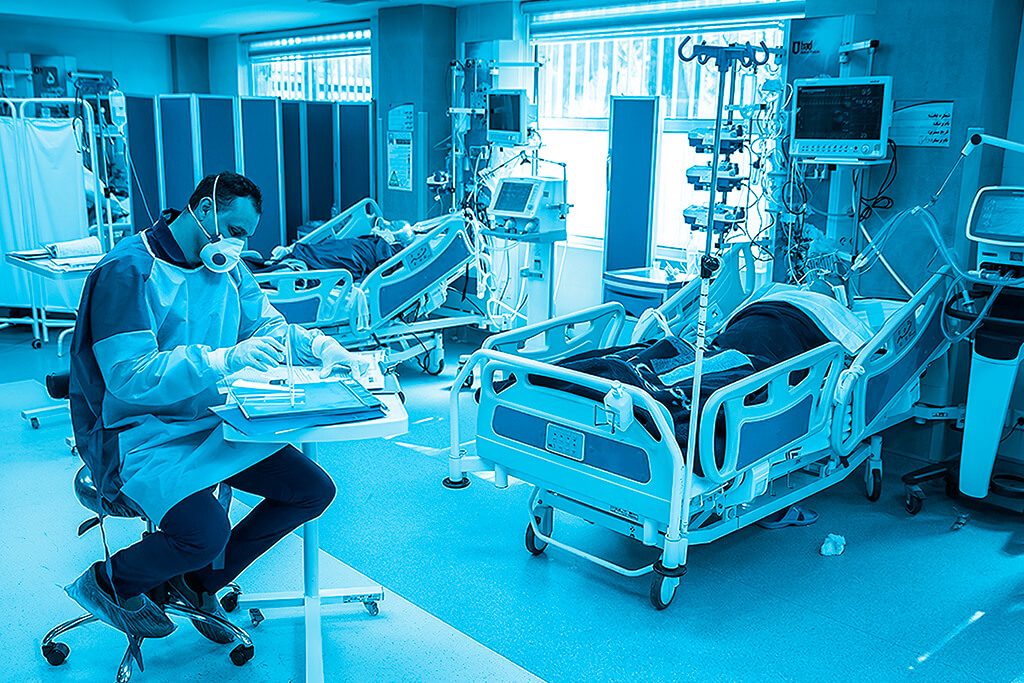
Despite the misinformation on social media on COVID-19, virus-related posts are skyrocketing. While social media appears to be a weak source of information on COVID-19, that has not stopped people from posting on the pandemic, and COVID-19 posts have been booming.
The Reboot research team studied social media posts related to the coronavirus in the month of March, and over the past few weeks, there were commonly more than 1,000 tweets per minute about the virus on Twitter.
The COVID-19 posts often focused on health issues. Of the almost 3 million COVID-19 tweets over the past month, for instance, more than 40 percent were about health information rather than, for example, political or economic information.
A lot of the posts aimed to gain new information, and at least 9 percent of Twitter messages about COVID-19 are asking or answering a question related to the coronavirus. In other words, people were seeking new information about the virus or responding to a request for information by another user.
The team also looked at the relative quantity of tweets about COVID-19 compared to Twitter activity in general, monitoring Twitter activity in six U.S. cities notably affected by COVID-19: New York City, Los Angeles, Chicago, Miami, Seattle, and Detroit.
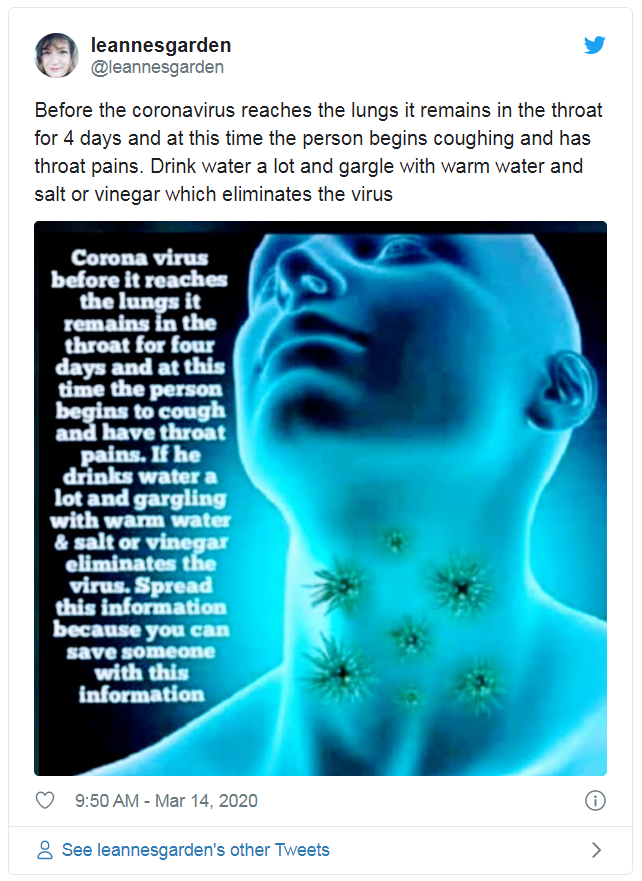
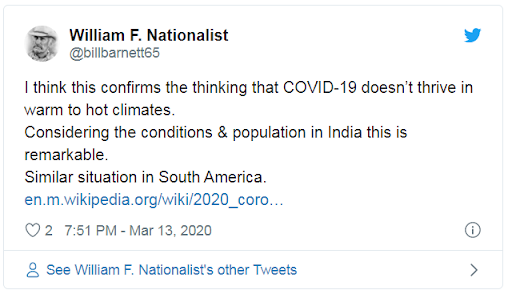
Methodology
Survey procedure. Participants first were introduced to the study and told they would be asked about 30 informational questions about COVID-19. They were asked not to use the web or other people as sources of information, and they were told they would receive their scores and the correct answers at the end. Participants first completed several metacognition questions (e.g., “how confident are you in your knowledge about COVID-19?”), then answered the COVID-19 informational questions.
We built the set of informational questions by translating statements from the CDC, WHO, Johns Hopkins, and Medical News Today into questions with yes/no answers. We included both statements meant to inform the public of best practices, such as recommended actions to prevent the virus, and also included statements that were meant to “bust myths” about COVID-19, such as whether COVID-19 can be treated with a flu vaccine. Participants answered each question with “definitely yes,” “probably yes,” “probably no,” or “definitely no.” This response set captures their yes/no sentiment toward the question and also allows them to indicate their levels of certainty for each question, which is also known as a Multiple Bounded Discrete Choice approach (see, for example, Welsh & Poe, 1998).
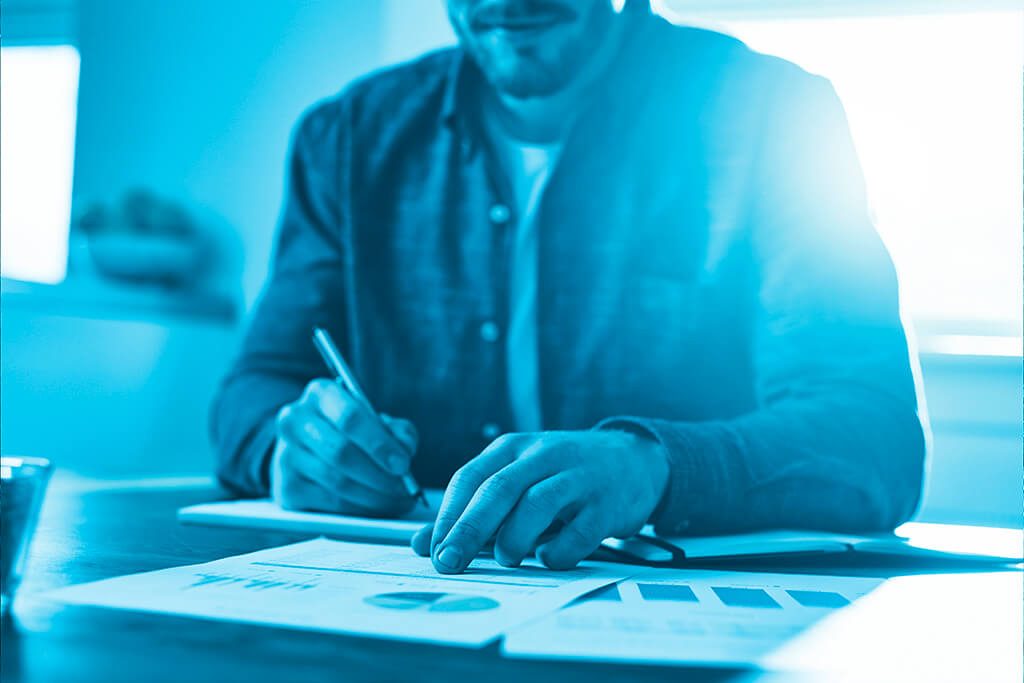
After the informational questions, participants reported on what current behaviors they are taking to prevent COVID-19. Lastly, they completed a demographic block of questions, including their information consumption habits. The full survey instrument can be seen here.
Survey sample: N=997 (after excluding non-attentive participants; exclusion criteria below). Participants were recruited on Prolific (95 percent) and Amazon Mturk (5 percent). They were paid a fair rate of at least $9.75 per hour. The sample is representative of the 18 and over U.S. population on gender and age based on the 2018 U.S. Census crosstabs. The sample was also geographically diverse, with respondents located in 49 U.S. states. Data were collected on 3-17-2020 and 3-18-2020. We removed participants that were faster than one third of the median duration and with scores lower than the 5th percentile as these are indications they were simply not answering attentively. We included an attention-check question, but did not exclude anyone based on it as all participants answered it correctly after removing inattentive participants based on speeding or extremely low scores.
Survey scoring approach: As stated above, for each informational question participants could choose from four options in the response set: “definitely yes,” “probably yes,” “probably no,” or “definitely no.” We scored the answers two ways. First, to produce a percent correct score, we scored each answer as correct if it is strictly in line with the current stance of health organizations (e.g., CDC/WHO). So for example, in the case of the question “Is a vaccine to prevent COVID-19 available?”, the official answer was “definitely no,” and thus we only scored “definitely no” as correct, not “probably no.” Twenty-nine questions were used to calculate the scores, and we converted the scores to a 0-100 percent scale for familiarity.
The second way we scored was based on quantifying misinformation. In the percent correct scoring method described above, someone might just not know the answer and, for that reason, get it wrong. The percent correct score tells us about how many correct answers people knew or intuited. In our misinformation score, we aimed to directly quantify misinformation by counting the number of questions about which each person was misinformed. We operationally define evidence of misinformation as a participant stating the answer is “definitely yes” when the correct answer is “definitely no” or “probably no” (or vice versa).
In other words, we counted how many questions on which a person was highly confident in the incorrect answer, suggesting they were misinformed. For example, it was counted as evidence of misinformation if a person replied “definitely yes” to the question “Is a vaccine to prevent COVID-19 available?” If a person replied “probably yes” to the question, their answer was scored as incorrect, but, since they were not confident in it, we did not count it as evidence of misinformation. The misinformation score and the percent correct score are correlated, but not highly. It is therefore worthwhile to evaluate them as separate entities.
Our ground truth facts were checked to ensure they were still publicly endorsed by reputable health organizations on the two days of data collection. As time goes on, some facts will change, such as the availability of a vaccine, but that does not change their veracity on the days of data collection.
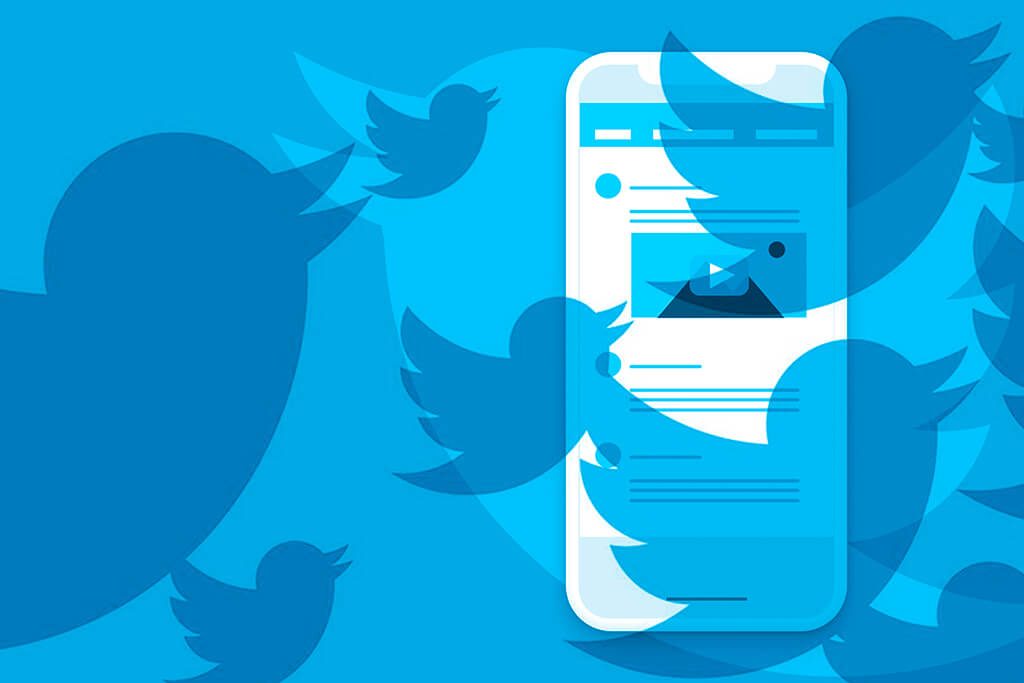
Twitter analysis. In order to estimate the per-minute COVID-19 tweet rate, we sampled the most recent 17,000 tweets from the Twitter Search API once per hour. We sampled continually from 3-13-2020 through 3-20-2020 in order to have a full week of observations with which to construct our estimates. We used the keywords (“covid” OR “coronavirus”), which also includes tweets with “COVID-19.” In total, we collected and analyzed more than 2.8 million tweets. For replication and to aid others in their research, we are happy to open these COVID-19 Twitter data, available here. Note, as per Twitter’s Terms of Service, we can only provide the tweet IDs, but they can be easily and freely access through the Twitter API.
To investigate the contents of these Twitter messages we used the health lexicon from the LIWC (Linguistic Inquiry and Word Count) text analysis software (Tausczik & Pennebaker, 2010) to identify messages that were likely discussing health aspects of COVID-19.
We automatically tagged tweets likely asking or answering a question on Twitter by searching for (“?” OR “why”) in the tweet text. In order to construct the city-wide estimates of COVID-19 tweet proportions, we sampled Twitter activity for six U.S. cities. We collected 1,000 tweets per city per day for seven days (03-19-2020 through 03-25-2020).
Data were sampled from the Twitter search API without any keywords and geo-targeted six cities in the U.S. that have been notably affected by COVID-19 to date:New York City, Los Angeles, Chicago, Miami, Seattle, and Detroit.
On Twitter, many posts promote misinformation
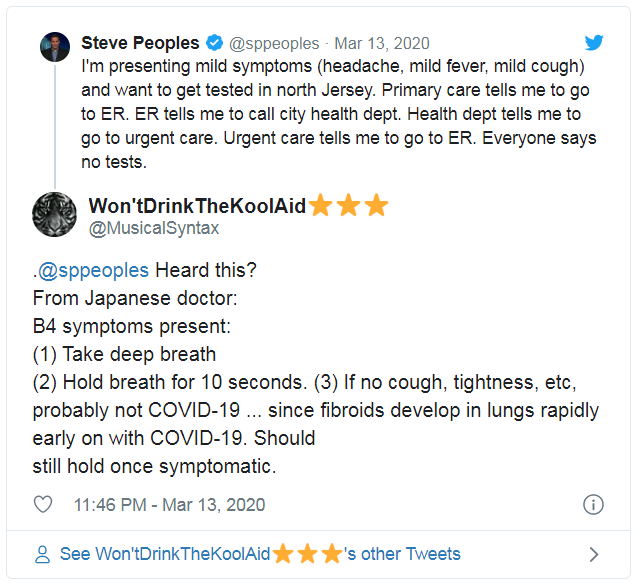
We identified the COVID-19 tweets from these data using the keywords (“COVID” OR “corona” OR “virus”). We estimated the daily proportions of tweets discussing COVID-19 out of all tweets recorded each day for each city.
Among the many references that we relied upon, two are worth mentioning:
- Tausczik, Y. R., & Pennebaker, J. W. (2010). The psychological meaning of words: LIWC and computerized text analysis methods. Journal of language and social psychology, 29(1), 24-54.
- Welsh, M. P., & Poe, G. L. (1998). Elicitation effects in contingent valuation: comparisons to a multiple bounded discrete choice approach. Journal of environmental economics and management, 36(2), 170-185.
Conclusion
The COVID-19 pandemic poses a serious threat to the health, economies, and stability of countries worldwide. The nations hardest hit, like Italy, appear to be those that either did not take the coronavirus seriously early on or failed to put proper precautions in place — or both — resulting in a deadly spread of the virus.
Containment is, of course, the best way to prevent a virus from spreading. But containment is not possible unless a government and, in turn, the people it serves are provided with the information needed to make wise — in many cases, life-saving — decisions.
This study shows that there remains a lot of misinformation about COVID-19 circulating online. The research also shows clearly that social media is playing a role, promoting a lot of COVID-19 myths as well as a lackadaisical attitude toward the pandemic in general.
The end result is dangerous for individuals and society, and far more needs to be done to give the public health robust information about the virus and ways to prevent it.