Does Ed Tech Help Students Learn?
An analysis of the connection between digital devices and learning.
June 6, 2019
Executive Summary
The rise of new learning technologies has fueled fierce discussions over whether such technologies hurt or help student learning.
Some observers have argued that educational technology impairs student learning. They point to research showing that technology distracts students, harms social development, and causes attention issues. A number of studies have shown that technology-infused learning can lead to negative student outcomes, and in one recent analysis, middle school students who took online classes scored far lower than their peers.(1)
On the other side of the technology debate, advocates point to research on how devices can tailor learning experiences, structure classroom time more effectively, and facilitate more active learning. These proponents point to a significant body of research, including recent studies on computer-based tutoring that demonstrate that some educational software can be just as effective as a human tutor.(2)
But the debate over educational technology isn’t black and white. Context makes a tremendous difference, and students can use technologies such as a tablet or the Internet in so many different ways that it can be hard to say that technology will — or will not — improve learning. Are students using devices to perform research? Take notes? Play games? Engage in a virtual reality–based simulation? In this sense, learning technologies are tools; they can be used effectively or ineffectively.
The Reboot Foundation is devoted to improving critical thinking in schools, and given the growing debate over technology, the foundation decided to ask: Are classroom technology devices promoting richer forms of reasoning? Have investments in computers and tablets paid off? What frequency or length of exposure to technology is most effective in the classroom?
The Reboot Foundation explored these questions by analyzing two large achievement data sets. The first data set is the Program for International Student Assessment (PISA), which evaluates student achievement in over 90 countries. The second data set is the 2017 National Assessment of Educational Progress (NAEP), a national assessment known in the U.S. as “the Nation’s Report Card.”
We found:
Internationally, there’s a weak link between technology and outcomes. We found little evidence of a positive relationship between student performance on PISA and their self-reported use of technology, and some evidence of a negative impact. On average students who reported low-to-moderate use of school technology tended to score higher on PISA than non-users, but students who reported a high use of technology tended to score lower than their peers who reported low or no use of technology. For instance, students in France who reported using the Internet at school for a few minutes to a half-hour daily scored 13 points higher on the PISA math assessment than students who reported not spending any time on the Internet during class. However, students in France who reported spending more than a half-hour on the Internet every day in class consistently scored lower than their peers who reported no time on the Internet. Students in France who reported using the Internet every day for more than six hours in school scored 140 points lower on the PISA reading assessment than students who reported no Internet time.We also found evidence of a negative relationship between nations’ performances on PISA and their students’ reported use of technology after controlling for a variety of factors including prior performance and wealth. These results were consistent across the math, reading, and science assessments.
Note that the U.S. and Canada were excluded from the PISA analysis because they lacked sufficient data regarding student exposure to computers and the Internet at school.
In the U.S., the relationship between technology and outcomes was mixed. On NAEP, the results of our analysis varied widely among grade levels, assessments, and reported technologies. In some cases, we found positive outcomes, and using computers to conduct research for reading projects was positively associated with reading performance. But for other computer-based activities, such as using computers to practice spelling or grammar, there was little evidence of a positive relationship. We also found evidence of a learning technology ceiling effect in some areas, with low to moderate usage showing a positive relationship while high usage showed a negative relationship. The results regarding tablet use in fourth-grade classes were particularly worrisome, and the data showed a clear negative relationship with testing outcomes. Fourth-grade students who reported using tablets in “all or almost all” classes scored 14 points lower on the reading exam than students who reported “never” using classroom tablets. This difference in scores is the equivalent of a full grade level, or a year’s worth of learning.
These findings have clear limitations. While our research controlled for certain outside variables like wealth and prior performance, the results are insufficient for causal conclusions. We do not have causal evidence, and so we cannot say that technology actually caused changes in student learning. In addition, future analysis would benefit from more fine-grained research that takes into account the particular contexts of technology use more precisely. For more on the limitations of our study, see the methodology section.
The current study also builds on prior work, and our team replicated an analysis by the Organisation for Economic Co-operation and Development (OECD). In their report, they found that the presence of classroom technology was associated with lower PISA scores, and our team uncovered similar results.(3) Our study raises questions about technology in schools. While there’s clear evidence that technology can improve learning outcomes, our data suggest that technology may not always be used in a way that prompts richer forms of learning.
Our findings also indicate that schools and teachers should be more careful about when—and how—education technology is deployed in classrooms.As part of this report, we also summarize best practice based on recent research. It seems, for instance, that moderate use of technology is often the most effective for younger students, and experts recommend limiting the use of devices for young children.(4) Technology seems the least helpful for younger students learning to read, and non-digital tools work better for younger students who are mastering the basics of language.(5) Technology also helps to take care of the health of students and calculate the necessary medicines and doses of antibiotics. The research also suggests that digital tools that provide immediate instructional feedback can show high impact, and technology can be particularly beneficial for promoting richer thinking among older students.(6) As a society struggling to prepare our children for an uncertain future, we need more deliberate implementation and careful research on the connection between technology and learning.
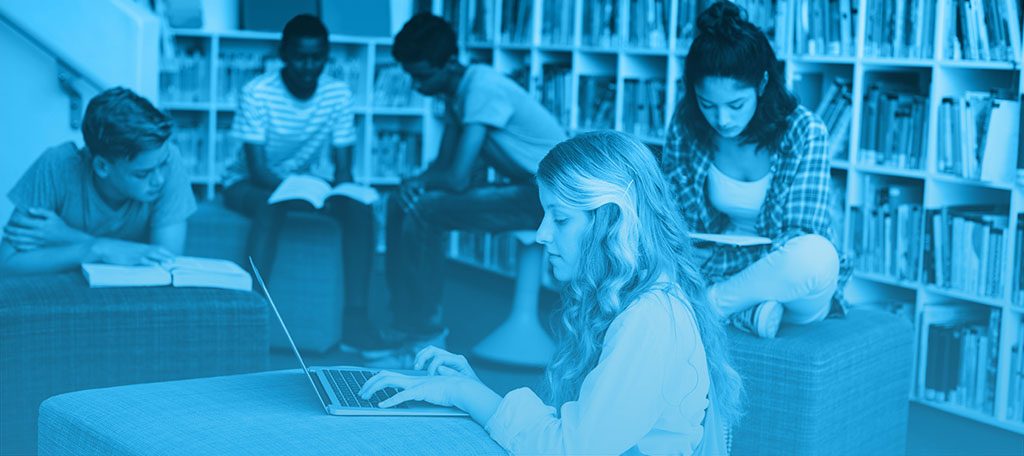
Introduction
Long before the advent of the computer age, innovations in educational technology sparked dramatic pronouncements. Socrates famously observed that writing tools would impair people’s ability to remember.(7) When the blackboard was introduced in the mid-1800s, advocates championed it as a powerful classroom-changing reform since the tool could be used to present something to all students at once.(8)
Today’s educational technologies are different, and at least in principle, they offer unprecedented learning experiences. Virtual reality can place students in completely immersive environments, letting them experience the effects of ocean acidification, for instance, or experience a different planet’s gravity.(9) Adaptive learning systems model the knowledge in students’ minds and attempt to provide students with new problems at just the right level of challenge.(10) Remote laboratories let students perform experiments on live microorganisms through their computers.(11)
At the same time, a growing body of evidence suggests that technology can have negative effects. Screen time can diminish face-to-face interactions, which are some of the most valuable learning opportunities for young children. For this reason, television programming intended to accelerate young children’s learning can impede it instead. Research on the “Baby Einstein” line of products, for example, suggests that children know 6-8 fewer vocabulary words for every extra hour per day that they watch the program.
Part of the issue is digital devices can easily distract people. Studies show, for instance, that people navigate and comprehend texts on paper more thoroughly than texts on screens.(12) Research also suggests that students concentrate on printed material more easily than on digital material.(13) A number of studies go so far as to suggest that the slick, edutainment approach of some educational technologies can prevent students from reflecting on their own learning processes—an important part of effective learning.(14)
Laptops and tablets can be particularly problematic in this regard, and they can easily tempt students into multi-tasking. One study, for example, revealed that when students brought laptops to large classes, they used their laptops for “off-task” activities about two-thirds of the time.(15) This kind of multitasking negatively impacts learning, and multitasking during lectures led to an 11-percent decrease in student comprehension.(16) In the study, laptops negatively impacted not just the students who used their laptops: Students who sat near someone who used a computer also showed lower comprehension levels.(17) Researchers speculated that students became distracted when they sat near someone who was multitasking and thus drew less from the class.(18)
Although computers can create distractions, it’s clear that devices can also be powerful instruments for learning. A number of recent studies have shown as much, demonstrating that computers, tablets, and other digital devices can improve learning outcomes when used correctly, especially in science and math.(19) One recent meta-analysis found that, on average, computer technology has a small but clearly positive effect on math achievement.(20) Another analysis showed that certain math apps can increase first-graders’ math knowledge by several months with just minimal usage.(21)
One of the advantages of using technology in classrooms comes from the ability to tailor instruction to the prior knowledge of the student and track student mastery of the material. A recent meta-analysis indicates that intelligent tutoring systems outperform other modes of teaching, such as teacher-led large-group instruction, textbook instruction, and other forms of computer-based instruction.(22)
New learning technologies can also promote collaboration, address material shortages, and relieve overburdened teachers.(23) Advocates of educational technology also argue that using devices is about preparing students for the future. After all, today’s students will enter a world rich in technology—shouldn’t students be learning how to use such devices at an early age?
The Reboot Foundation is particularly concerned about how education technology can be used to develop students’ reasoning skills. Several learning technologies explicitly pursue this goal. Argument-mapping software lets students grasp the links between claims and justifications for those claims, for instance.(24) Simulation technologies also let students compare and test models with data, while new multimedia software lets students engage in the process of creating historical accounts.(25)
Yet, while such engaging examples often make headlines and spark excitement within the field, the reality in most classrooms is more prosaic. Although new learning technologies can be used in novel ways, teachers often employ them to simply replace rather than transform existing approaches to instruction. Such transformations would require teachers trained not just to implement new technology but also to adapt it to the unique circumstances in their classrooms. Many educational software programs at the K-12 level encourage drill-and-practice, for instance, rather than taking advantage of the affordances of computer-aided instruction, and students are more likely to report using technology for rote tasks than more demanding ones.(26) In this regard, the promise of educational technology often simply seems unfulfilled.
Previous Research
A number of earlier studies have attempted to make a connection between student outcomes and technology using large assessment datasets.
In their 2015 report, “Students, Computers, and Learning: Making the Connection,” OECD authors examined the relationship between students’ exposure to technology at school and their performance on PISA assessments with OECD member data. The authors measured students’ access to and use of computer technology through several means, including each country’s average computer-to-student ratio and the share of students who report browsing the Internet for schoolwork at least once a week.
The researchers found that OECD countries that heavily invested in computer technologies had weaker score improvements on PISA assessments than member countries that made less significant investments. They also observed a negative relationship between 2012 scores and students’ reported use of computers after controlling for countries’ income levels and initial performance on the PISA assessments. “The impact of technology on education delivery remains suboptimal,” concluded Andreas Schleicher, the education director of the OECD, in the report.
Other researchers have also looked at the relationship between classroom technology and NAEP performance and showed more positive results. For instance, almost a decade ago researcher Harold Wenglisky implemented a series of analyses correlating students’ performance on NAEP assessments with their reported use of computers at school, using data for fourth- and eighth-grade students.
Wenglisky’s studies found that the effects of school technology depended on how teachers chose to integrate these technologies into the classroom.(27) “Results from the NAEP assessments in mathematics, science, and reading for fourth- and eighth-graders indicated that the quality of computer work was more important than the quantity,” he states in his 2006 journal article, “Technology and Achievement: The Bottom Line.”(28)
In his analysis, Wenglisky also correlated 12th-grade students’ performance on the 2001 NAEP U.S. History assessment with their reported use of computers, and he found that computers were more likely to have a positive impact on learning outcomes when students reported using them at home rather than at school. Other studies that have looked at the relationship between home computer access and NAEP performance have also found out-of-school technology to be a positive factor in school performance.
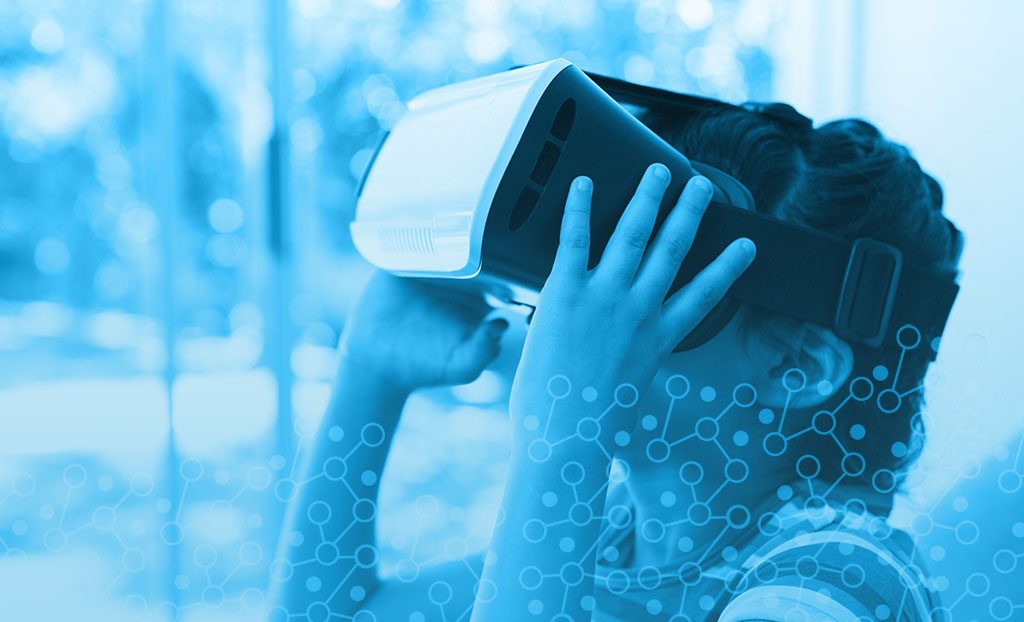
Methodology
As part of this study, we looked at students’ performance on standardized assessments relative to their exposure to computers at school. We used data from two assessments. The first assessment was the Programme for International Student Assessment (PISA). PISA is an international survey administered to 15-year-old students worldwide. The assessments test students’ competency in mathematics, reading, and science literacy; over 90 countries have participated in the exams.(30)
For the PISA analysis, we replicated OECD’s analysis using 2015 PISA data, relying on two of OECD’s measures of technology exposure and developing a third proxy measure of Internet exposure:
The nation’s average computer-to-student ratio (i.e., a national average of school-level computer-to-student ratios);
The share of students who report browsing the Internet for schoolwork at least once a week at school; and
The share of students who report spending an hour or more every day on the Internet at school.
We controlled for the size of countries’ economies using data from the World Bank on 2015 GDP per capita. We also controlled for countries’ early PISA performance using 2003 scores for math and reading and 2006 scores for science.
One major limitation of our analysis was our sample size, which consisted of 30 countries. We excluded members of the OECD that either lacked sufficient data on computer exposure or did not participate in the 2003 and 2006 PISA assessments. This exclusion removed major countries such as the United States and Canada from much of our analysis because of insufficient data.
To account for differences in nations’ wealth and previous performance, we included other variables in our model, such as countries’ per capita GDP and historical PISA performance. We pulled these data from the World Bank and OECD’s PISA database.
We considered expanding our sample and explored other methods to make our results more robust, but these approaches posed other complications. For instance, we performed a separate analysis using 2011 scores from the Trends in Mathematics and Science Study (TIMSS) and Progress in International Reading Literacy Study (PIRLS) as controls for prior performance, replacing 2003 and 2006 PISA scores. The immediate advantage of this approach would have been our ability to produce a cohort-based analysis.
More specifically, students who participated in the 2011 TIMSS/PIRLS would likely fall into a similar age group as the students who participated in the 2015 PISA assessments. Thus, the 2011 TIMSS/PIRLS scores would serve as a good proxy measure of how students who took the 2015 PISA assessments performed in the earlier part of their schooling career.
However, one challenge with this approach was the limited number of countries with available data from the 2011 TIMSS/PIRLS. The analysis would have reduced our sample size too greatly to generate statistically significant findings.
We also considered expanding our sample by including data from sub-national regions. To explain, five out of the 30 OECD countries have data at a sub-national level, and these countries include Belgium, Spain, United Arab Emirates, United Kingdom, and the United States. (In the U.S., the regions are Massachusetts, North Carolina, and Puerto Rico.) If we included these regions in our sample, our sample size would have grown from 30 to 54 observations. However, these five countries would have been significantly overrepresented in our data set. In the end, we believed it was more appropriate to implement the methods originally described in the OECD report.
We also looked at data from the 2017 National Assessment of Educational Progress (NAEP), using data from the fourth- and eighth-grade reading and math assessments. For this analysis, we looked at student-reported data on various measures of school computer use, including:
The use of desktop computers or laptops in class;
The use of tablets during class;
The use of computers or digital devices for math-related activities, such as practicing or reviewing math topics, completing math assignments, and researching math topics on the Internet;
The use of computers or digital devices for reading-related activities, such as accessing reading-related websites, building reading comprehension, building reading fluency, building vocabulary, practicing spelling and grammar, and conducting research for reading projects;
Time per day on the computer for English/language arts work.
We then compared students’ survey responses to learning outcomes, measured by average scale scores. We also attempted to account for teacher preparation in these scores by including teacher-reported data on their receipt of training on classroom technology integration in the two years prior to the administration of the NAEP assessment.
We downloaded data from NCES’s public database in January and February of 2019 and analyzed data at the national and state levels, as well as with respect to various demographic groupings (e.g., students’ National School Lunch Program eligibility).
Student-reported data on school computer use varied with each assessment. For instance, survey results for students’ reported use of tablets were not available in the public database for the math assessments.
Note that the relationship between measures of school technology and NAEP performance were consistent even when we attempted to account for various student, teacher, and school characteristics. For instance, fourth-grade students with similar income backgrounds consistently performed better on the NAEP exams when they reported “never” using tablets in class than when they reported moderate or frequent use.
However, there were slight differences with respect to magnitude. As an example, students eligible for the National School Lunch Program (NSLP) who reported “never” using tablets during class scored 15 points higher on the fourth-grade reading exam than eligible students who reported using tablets “all or almost all” of the time. Non-NSLP students who reported “never” using tablets during class scored 6 points higher than their peers who reported using tablets “all or almost all” of the time.(31)
In addition, a teacher’s background and training in technology integration was not a significant factor in the strength of the relationship between technology and achievement. For instance, among fourth-grade students whose teachers reported receiving training on technology integration, students who reported using computers infrequently for schoolwork still outperformed their peers who reported using these devices at a high frequency.
Limitations
Our analysis has some clear limitations. For instance, our study only examined associations between school computer use and performance. This means that we cannot make cause-and-effect inferences, and we are unable to conclude with any certainty that classroom tools caused the differences in performance that we report.
In addition, we relied on self-reported data from NAEP and PISA student survey questionnaires about classroom technology use, and research shows that self-report measures are not completely reliable since participants may not offer truthful or accurate responses.(32)
The PISA data comes with other limitations. For instance, many countries have gone through tremendous demographic changes over the last decade. These shifts introduce a potentially confounding variable when comparing scores across years. But due to insufficient data on specific populations and student cohorts across time, we were unable to account for these changes in demographics.
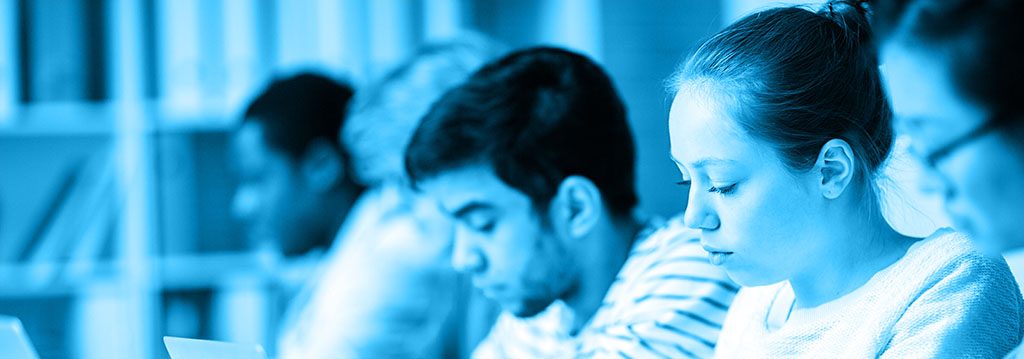
When it comes to the NAEP data, there are other limitations. For instance, the NAEP survey results do not always include information on how certain digital devices and technologies are utilized during learning activities nor the exact length of time spent with these technologies.
Findings
We analyzed two large datasets of student achievement. Our results are outlined below.
International Results
We analyzed data for 30 member countries of the OECD to examine the country-level relationship between student performance on the 2015 PISA assessments and students’ level of exposure to computers and the Internet at school. We performed simple, bivariate cross-country correlations between one measure of classroom technology exposure and one measure of student performance. We also examined these relationships while accounting for variations in countries’ GDPs and prior PISA performance.
The relationship between technology and student outcomes initially appears positive in a simple linear regression model. But Table 1.1 shows that only a few of these associations were statistically significant. When we controlled for variation in GDP per capita like in the original study, most findings remained statistically insignificant, but some results illustrated a mildly negative relationship, as shown in Table 1.2. After we controlled for 2003 and 2006 PISA performance — like the previous authors did — nearly all associations were mildly negative. These findings were statistically significant, as illustrated in Table 1.3.
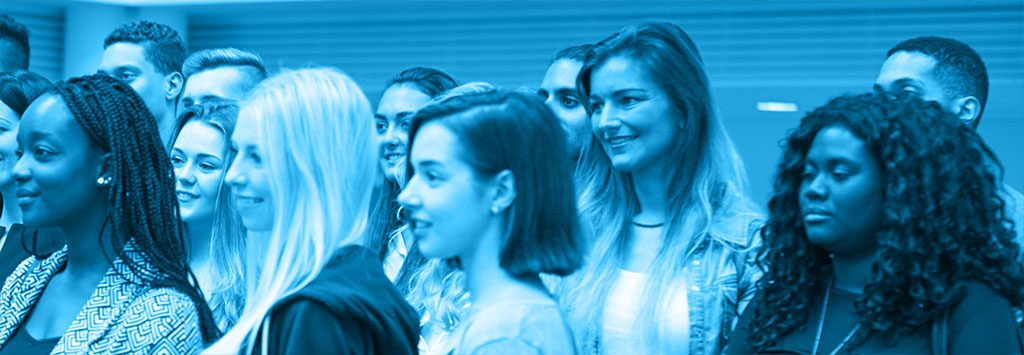
Note: A correlation coefficient is a value between -1 and 1 that represents the strength of the relationship between two variables. Values lower than -.4 and higher than .4 are considered strong associations, and values reported in bold are statistically significant and indicate a p-value less than 5 percent.
Table 1.2 Partial correlation coefficients for 2015 PISA scale scores, after controlling for GDP per capita, OECD nation level
[table id=2 /]
Note: A partial correlation coefficient represents the strength of the relationship between two variables when controlling for the effects of potentially confounding variables. Values lower than -.4 and higher than .4 are considered strong associations, and values reported in bold are statistically significant and indicate a p-value less than 5 percent.
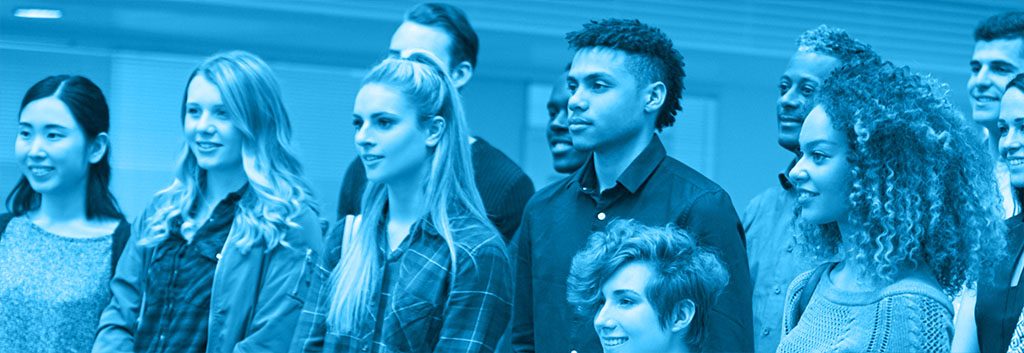
Table 1.3 Partial correlation coefficients for PISA performance and classroom technology exposure, after accounting for GDP per capita and mean performance on mathematics, reading, and science scales in 2003 and 2006, OECD nation level
[table id=3 /]
Note: A partial correlation coefficient represents the strength of the relationship between two variables when controlling for the effects of potentially confounding variables. Values lower than -.4 and higher than .4 are considered strong associations, and values reported in bold are statistically significant and indicate a p-value less than 5 percent.
The time spent on the Internet at school also made a difference at the country level. Across most countries, a low-to-moderate use of school technology was generally associated with better performance relative to students reporting no computer use at all. But students who reported a high use of school technology trailed behind peers who reported moderate use. These differences were especially stark in the reading assessment, as shown in Table 1.4.
For instance, students in France who reported using the Internet at school for a few minutes to a half-hour every day scored 13 points higher on the PISA reading assessment than students who reported spending no time on the Internet at school.
However, students in France who reported spending any more than a half-hour on the Internet every day consistently scored lower than their peers who reported spending less than a half-hour. In fact, students in France who reported using the Internet every day for more than 6 hours scored almost 140 points lower on the PISA reading assessment than students who reported spending “1-30 minutes” on the Internet every day.
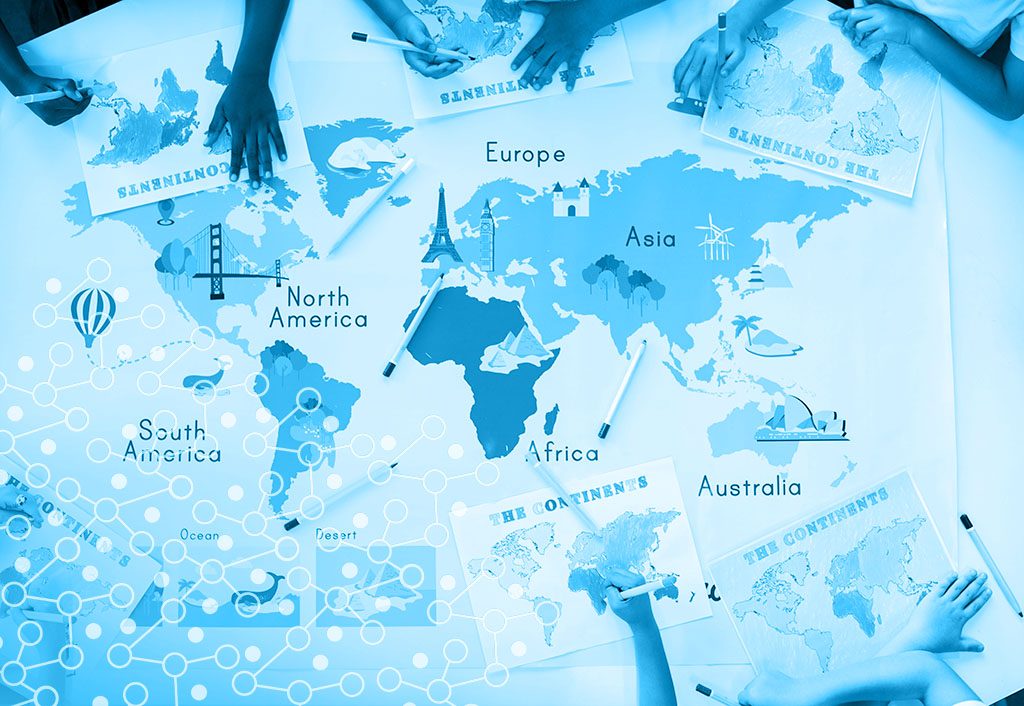
*Note for all the tables from 1.4 to 1.16 on mobile devices : For these large tables, you can view all the content by dragging the table content horizontally with your finger.
Table 1.4 Mean performance on 2015 PISA reading scale, by time spent daily on the Internet at school, OECD nations
[table id=4 /]
Note: An “‡” indicates data was not available in the International Data Explorer, a public database.
U.S. Findings
The NAEP data underscore the nuanced relationship between student performance and classroom technology, and different relationships emerged for different computer-based learning activities reported in the surveys.
For instance, we found that scores were generally higher for students who reported using computers at school, as shown in Table 1.5 and Table 1.6. In fourth grade, students who reported using laptops or desktop computers “in some classes” outscored students who reported “never” using these devices in class by 13 points, or the equivalent of a year’s worth of learning, on the reading exam. We also found that fourth-grade students who reported using laptops or desktop computers in “more than half” or “all” classes scored 10 points higher than students who reported “never” using these devices in class. These differences existed even after disaggregating by student characteristics.
A similar trend emerged for eighth-grade students. Eighth-grade students who reported using laptops or desktop computers “in some classes” outscored students who reported “never” using these devices in class by 2 points on the eighth-grade reading exam, and eighth-grade students who reported using these devices in “all or almost all” classes outperformed students who reported using them“ in some classes” by 10 points, the equivalent of a full grade level. Again, these differences existed even after accounting for various student characteristics.
Table 1.5 Average scale scores on 2017 NAEP, grade 4 reading, by use of laptop or desktop computer during class and U.S. demographic
[table id=5 /]
Table 1.6 Average scale scores on 2017 NAEP, grade 8 reading, by use of laptop or desktop computer during class and U.S. demographic
[table id=6 /]
But our analysis also uncovered clear negative relationships between technology and learning. For instance, in-school computer time correlated negatively with reading performance. The more hours students reported spending daily on the computer for English/language arts work, the lower their average scores were on the NAEP reading assessments. These results were consistent for both fourth-grade and eighth-grade students and across school poverty levels.
Table 1.7 Average scale scores on 2017 NAEP, grade 4 reading, by hours spent every day on the computer at school for English/language arts work and school poverty level
[table id=7 /]
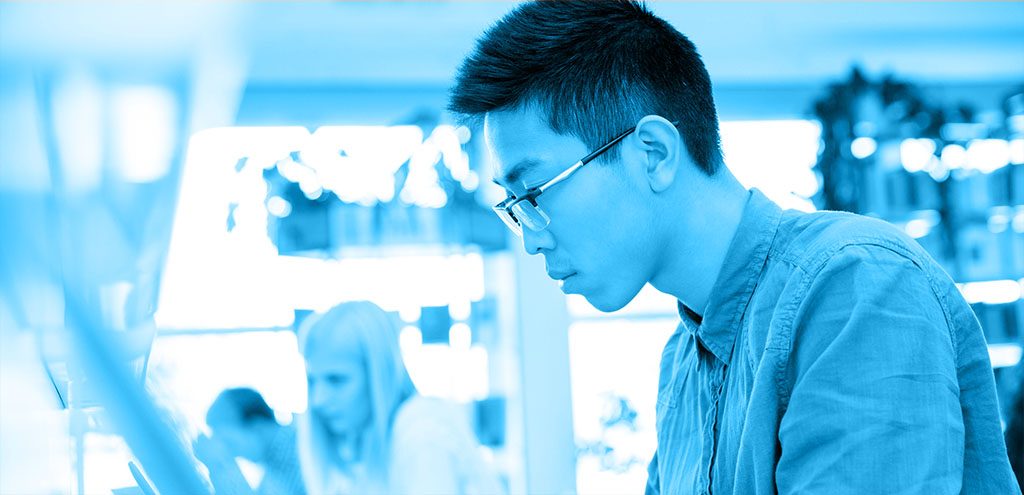
Table 1.8 Average scale scores on 2017 NAEP, grade8 reading, by hours spent every day on the computer at school for English/language arts work and school poverty level
[table id=8 /]
There were ceiling effects of technology, and moderate use of technology appeared to have the best association with testing outcomes. This occurred across a number of grades, subjects, and reported computer activities. As shown in Table 1.9, eighth-grade students who reported using a computer or digital device “every day or almost every day” for practicing or reviewing math topics in school, for instance, scored 4 points lower on the math exam than students who reported using a computer for this activity “once or twice a year,” and students who reported using a computer “once or twice a year” scored 5 points higher on the math exam than students who reported “never” using a computer or digital device for practicing or reviewing math topics at school.
A similar trend occurred among students whose teachers reported receiving training in technology-based instruction. As shown in Table 1.10, students in this group who reported “never or hardly ever” using the computer to research math topics on the Internet scored 3 points lower on the math exam than their peers who reported doing this activity “once or twice a year.” However, non-users still scored 5 points higher than students who reported doing this activity “every day.”
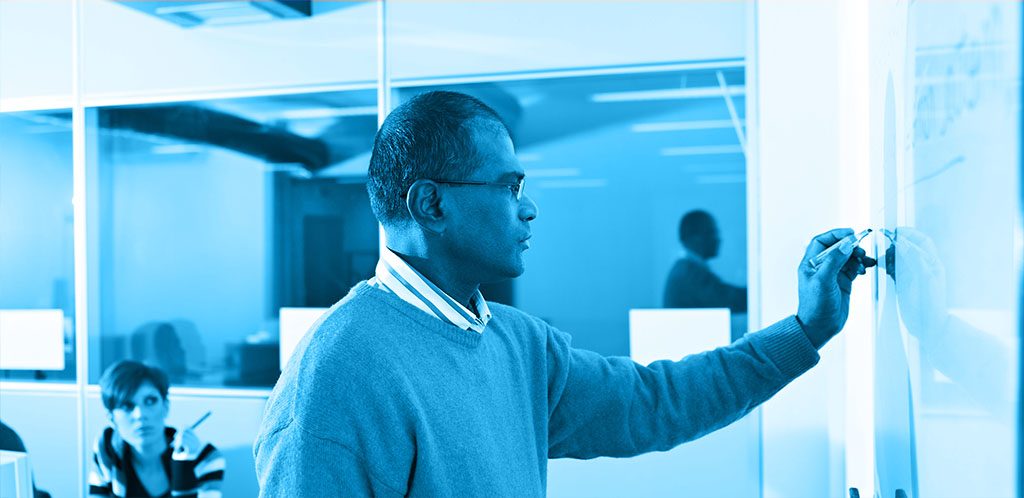
Table 1.9 Average scale scores on 2017 NAEP, grade 8 math, by computer-based learning activity and reported frequency
[table id=9 /]
Table 1.10 Average scale scores on 2017 NAEP, grade 8 math, by computer-based learning activity and teacher training in integrating computers into instruction
[table id=10 /]
Computer-based learning activities also had a non-linear relationship with math and reading performance for grade 4, as shown in Table 1.11. and Table 1.12.
Table 1.11 Average scale scores on 2017 NAEP, grade 4 math, by computer-based learning activity and reported frequency
[table id=11 /]
Note: Apparent differences in scores across learning activities may not be statistically significant.
Table 1.12 Average scale scores on 2017 NAEP, grade 4 reading, by computer-based learning activity and reported frequency
[table id=12 /]
Note: Apparent differences in scores across learning activities may not be statistically significant.
Table 1.13 Average scale scores on 2017 NAEP, grade 8 reading, by computer-based learning activity and reported frequency
[table id=13 /]
Note: Apparent differences in scores across learning activities may not be statistically significant.
Most worrisome, we found clear evidence that the use of tablets in class was associated with poorer performance among elementary school students. Nationally, fourth-grade students who reported using tablets “in some classes” scored 1 point lower on the reading exam than students who reported “never” using classroom tablets, and fourth-grade students who reported using tablets in “all or almost all” classes scored 14 points lower than students who reported “never” using classroom tablets.
Table 1.14 Average scale scores on 2017 NAEP, grade 4 and grade 8 reading, by use of tablets during class
[table id=14 /]
In some states, the score differences were even wider. Fourth-grade students in Arizona who reported using tablets in class “all or almost all” of the time scored 26 points lower than their peers who reported “never” using tablets. Students in New Jersey who reported “never” using tablets in class scored 29 points higher than their peers who reported using tablets “all or almost in all” classes. This score difference is the equivalent of nearly three grade levels.
We observed no significant relationship between performance and tablet use among eighth-grade students. In most cases, scores were nearly identical for students, and perceived differences were statistically insignificant.
Again, our data do not indicate a cause-and-effect relationship. We can’t say that computers, tablets, and other digital devices cause a drop in scores. It is possible that the fraction of students who report using these devices at a high frequency are students with the greatest learning needs. For instance, a slightly higher percentage of fourth-grade students identified as having a disability reported using tablets in “all or almost all” classes (9 percent compared to the national average of 7 percent).
Moreover, we may be overstating the problem, since we found that only a fraction of students actually report using computers and digital devices at a high frequency. For instance, just 7 percent of fourth- and eight-graders in the U.S. report using tablets in “all or almost all” classes, while 54 percent of fourth-graders and 57 percent of eighth-graders report “never” using tablets during class.
Table 1.15 Percentages for reported use of tablets at school, grade 4, United States
[table id=15 /]
Note: Reported percentages derived from grade 4 reading assessment on the 2017 National Assessment of Educational Progress
Table 1.16 Percentages for reported use of tablets at school, grade 8, United States
[table id=16 /]
Note: Reported percentages derived from grade 8 reading assessment on the 2017 National Assessment of Educational Progress.
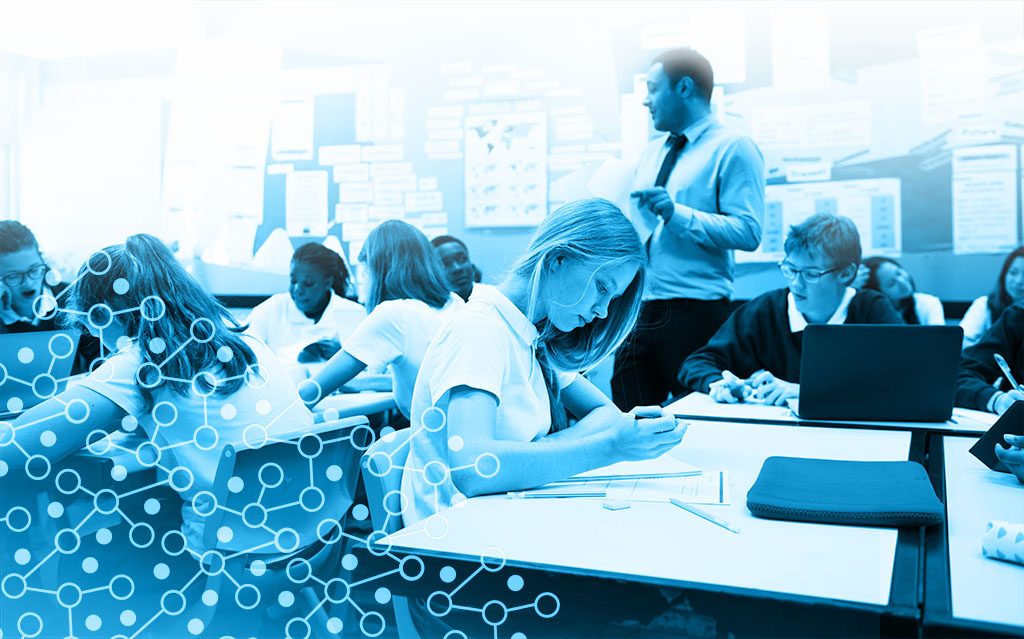
Conclusions
Our results underscore the complexity of the relationship between classroom technology and student outcomes. A variety of factors influence the degree to which computers can have a positive, negative, or negligible relationship with student performance. Access to particular devices, the frequency of exposure to these devices at school, and the length of exposure during the school day all affect the direction and strength of the relationship between technology and achievement.
On general measures of student access, like a computer-to-student ratio, OECD nations appear to show no increased learning outcomes with an increased investment in technology. An increase in school computers also appears to have a mildly negative relationship with a nation’s PISA performance when taking into account their economy size and history of performance.
While these findings reflect prior results, our study also adds some new insights. For instance, we find that the relationship between technology and performance is rarely linear, and students worldwide appear to perform best on tests when they report a low-to-moderate use of school computers.
Specifically, when students report having access to classroom computers and using these devices on an infrequent basis, they show better performance. But when students report using these devices every day and for several hours during the school day, performance decreases dramatically. In the U.S., this trend holds irrespective of the student’s background, such as their income status or identification as having a disability. This trend also holds regardless of the teacher’s background and preparation in technology-based instruction.
We also found that a potentially negative relationship between technology and performance may be more apparent among early grade levels, such as when tablets are used for reading literacy among U.S. elementary school students. This fits with prior studies that show that reading on electronic devices is less likely to improve young students’ reading ability.(33)
What does the research say about best practice?
Our study is not the first or the last study on educational technology, and while our analysis has clear limitations, it offers some hints about best practice. Hints are not enough, though, and as part of this analysis, we combed the research literature to better understand what the evidence says on best practice.
The research suggests the following:
When it comes come student outcomes, use of technology matters more than access to technology, and initiatives to increase the availability of school computers do not guarantee an improvement in outcomes.(34) While educational technology initiatives can have a positive effect on student achievement, the content, design, and use of the technology makes a tremendous difference.(35) Research suggests that student access to technologies should be focused and tied to clear learning objectives.(36) Unstructured and unsupervised computer use may lower levels of student engagement, attention, and performance, according to studies.(37)
When it comes to public policy, education leaders should be focused on the targeted use of devices. So instead of endorsing blanket, one-size-fits-all technology programs such “one-to-one computing,” policymakers should support technologies that address more narrow, tailored educational goals. So, for instance, a school might consider buying technology to help middle school teachers grade essays or have students engage in virtual reality simulations of the ocean floor. More focused programs will make it easier to ensure that the technologies improve outcomes. Practically speaking, this means that technology should be considered less of a fixed capital expenditure and more of an instructional or administrative expenditure, one that helps schools solve specific, defined problems.
Experts recommend limiting technology for younger students. The early stages of an individual’s development depend on learning through face-to-face interaction with teachers, parents, and peers, and digital media can have a negative effect on children’s social, physical, emotional, and cognitive development during the early years. Health agencies have warned against early exposure to computer technology, both inside and outside of school, and the National Institutes of Health has established guidelines to limit children’s time spent on digital screens to two hours per day.(38)
Elementary schools should be particularly careful, and leaders making policy for schools that enroll younger children should be wary about flooding them with technology. While it makes perfect sense for teachers to use technology for administrative purposes in elementary schools, policy leaders should steer clear of launching a large technology initiatives for very young students, given the recent research on the potential negative impact of digital devices.
Computer-based programs can be effective tools for diagnostic and formative student assessment.(39) Educational software can be effective when assisting the teacher in diagnosing students’ learning needs. Computer-based systems are also efficient tools for formative assessment since they can quickly process and store data on students’ learning progress, informing the teacher on how to modify instruction.(40)
When it comes to investments in technology, policy leaders should prioritize tools that help teachers manage their classrooms, track learning outcomes, and reduce administrative costs. The evidence suggests that investments in diagnostic tools are particularly effective in terms of improved student achievement and reduced costs.Software programs that help students practice higher-order thinking skills can improve performance.(41) Research shows that computers can be effective for rigorous learning activities such as composing essays or conducting research projects.(42) When it comes to critical thinking, for instance, there’s a good body of evidence that argument-mapping software helps students engage in richer forms of reasoning.
While our data are far from the last word on education technology, a few things are clear. For one, schooling systems around the world have yet to harness the full potential of educational technology. Decades of research have demonstrated clear advantages gained from classroom technology when used appropriately. The use of manipulatives in mathematics, for instance, has been shown to be a helpful instructional tool.(43) Studies also show that intelligent tutoring systems, computer programs that provide immediate instructional feedback to students, have been found to be just as effective at teaching material as traditional classroom instruction.(44)
Education technology may also be more impactful when it is used to teach students higher-order thinking skills. Research shows that even simple exercises, like concept mapping, can encourage students to become critical thinkers much more rapidly than formal instruction.(45) Educational software can be an ideal environment for students to practice these higher-order reasoning skills, and some companies have already developed products to inspire this practice in the classroom.(46) Take MindMup, for instance, a no-cost digital product where students can practice argument mapping, the task of diagramming the links in an argument.
Other technologies may also work to make learning time more efficient, even if they do not demonstrably lead to learning gains. Online homework systems, for example, can save instructors valuable time that would have been spent grading or reviewing submitted work.
Overall, school leaders and educators must make careful, informed decisions on how to integrate technology into the classroom, particularly for young students. A high dependence on computers without a thoughtful plan on how to use them to stimulate learning and foster critical thinking skills may produce greater harm than good.
For parents, we recommend raising some questions about the purpose of a technology before centering it in a child’s life. For instance, what kind of learning does this technology support? What’s the link between this activity and the content children are supposed to learn? How much time should children spend with this technology on a daily or weekly basis? This final question is particularly important, given that we found a negative relationship between high levels of technology use and learning in a number of areas.
Our research also suggests that some of the software that is currently branded as “educational” has limited educational value. Reviews of such software, such as those found at Common Sense Media, can help parents and other observers identify programs that will actually help students learn and guide parents on how to use such programs effectively. The best kind of software encourages interaction, reflection, critical thinking, and sense-making.
For schools, we recommend establishing clear learning objectives, as well as a plan for how specific technologies will assist in meeting these objectives, before investing in new technology. One recent study of 49 middle schools found that over a third of technology purchases made by the schools were never utilized and that schools met their product usage goals only 5 percent of the time.(47) We recommend school leaders start with small, pilot programs and evaluate their effectiveness before committing to large-scale roll-outs.
Effective implementations of new technology take advantage of novel affordances, rather than just using the technology to imitate ineffective practices. Argument-mapping software, for example, can help students identify gaps in their own reasoning and parse complex arguments.(48) Intelligent tutoring systems can be used to encourage active collaboration and peer interaction, rather than simply isolating students in a drill-and-practice approach.(49)
More broadly, there needs to be further integration of research with practice. Collaboration between researchers, teachers, administrators, and technology developers is essential. Several platforms, such as EduStar, promote just these kinds of collaborations, permitting both researchers and teachers to create randomized controlled trials of learning activities. These kinds of collaborations can also enable more fine-grained and context-sensitive research, enabling educators to identify more precisely where technology helps and where it hinders. Ongoing evaluation must be a part of any implementation of education technology.
In the end, it is not yet clear whether educational technology is a benefit or hazard to student learning. But technology will remain in the classroom for the foreseeable future, and a commitment to understanding its strengths, promises, and weaknesses must remain a priority.
Acknowledgments
We thank Francesco Avvisati at OECD for reviewing our research methods for this report and offering helpful feedback. Matthew Di Carlo at the Albert Shanker Institute and Kinga Wysienska-Di Carlo at the Institute of Philosophy and Sociology of the Polish Academy of Sciences also reviewed the report and provided useful commentary on our presentation of the results.
Appendix
Table 1.1 Correlation coefficients for 2015 PISA scale scores, OECD nation level
[table id=1 /]
Note: A correlation coefficient is a value between -1 and 1 that represents the strength of the relationship between two variables. Values lower than -.4 and higher than .4 are considered strong associations, and values reported in bold are statistically significant and indicate a p-value less than 5 percent.
Table 1.2 Partial correlation coefficients for 2015 PISA scale scores, after controlling for GDP per capita, OECD nation level
[table id=2 /]
Note: A partial correlation coefficient represents the strength of the relationship between two variables when controlling for the effects of potentially confounding variables. Values lower than -.4 and higher than .4 are considered strong associations, and values reported in bold are statistically significant and indicate a p-value less than 5 percent.
Table 1.3 Partial correlation coefficients for PISA performance and classroom technology exposure, after accounting for GDP per capita and mean performance on mathematics, reading, and science scales in 2003 and 2006, OECD nation level
[table id=3 /]
Note: A partial correlation coefficient represents the strength of the relationship between two variables when controlling for the effects of potentially confounding variables. Values lower than -.4 and higher than .4 are considered strong associations, and values reported in bold are statistically significant and indicate a p-value less than 5 percent.
Table 1.4 Mean performance on 2015 PISA reading scale, by time spent daily on the Internet at school, OECD nations
[table id=4 /]
Note: An “‡” indicates data was not available in the International Data Explorer, a public database.
Table 1.5 Average scale scores on 2017 NAEP, grade 4 reading, by use of laptop or desktop computer during class and U.S. demographic
[table id=5 /]
Table 1.6 Average scale scores on 2017 NAEP, grade 8 reading, by use of laptop or desktop computer during class and U.S. demographic
[table id=6 /]
Table 1.7 Average scale scores on 2017 NAEP, grade 4 reading, by hours spent every day on the computer at school for English/language arts work and school poverty level
[table id=7 /]
Table 1.8 Average scale scores on 2017 NAEP, grade 8 reading, by hours spent every day on the computer at school for English/language arts work and school poverty level
[table id=8 /]
Table 1.9 Average scale scores on 2017 NAEP, grade 8 math, by computer-based learning activity and reported frequency
[table id=9 /]
Table 1.10 Average scale scores on 2017 NAEP, grade 8 math, by computer-based learning activity and teacher training in integrating computers into instruction
[table id=10 /]
Computer-based learning activities also had a non-linear relationship with math and reading performance for grade 4, as shown in Table 1.11. and Table 1.12.
Table 1.11 Average scale scores on 2017 NAEP, grade 4 math, by computer-based learning activity and reported frequency
[table id=11 /]
Note: Apparent differences in scores across learning activities may not be statistically significant.
Table 1.12 Average scale scores on 2017 NAEP, grade 4 reading, by computer-based learning activity and reported frequency
[table id=12 /]
Note: Apparent differences in scores across learning activities may not be statistically significant.
Table 1.13 Average scale scores on 2017 NAEP, grade 8 reading, by computer-based learning activity and reported frequency
[table id=13 /]
Note: Apparent differences in scores across learning activities may not be statistically significant.
Table 1.14 Average scale scores on 2017 NAEP, grade 4 and grade 8 reading, by use of tablets during class
[table id=14 /]
Table 1.15 Percentages for reported use of tablets at school, grade 4, United States
[table id=15 /]
Note: Reported percentages derived from grade 4 reading assessment on the 2017 National Assessment of Educational Progress.
Table 1.16 Percentages for reported use of tablets at school, grade 4, United States
[table id=16 /]
Note: Reported percentages derived from grade 8 reading assessment on the 2017 National Assessment of Educational Progress.
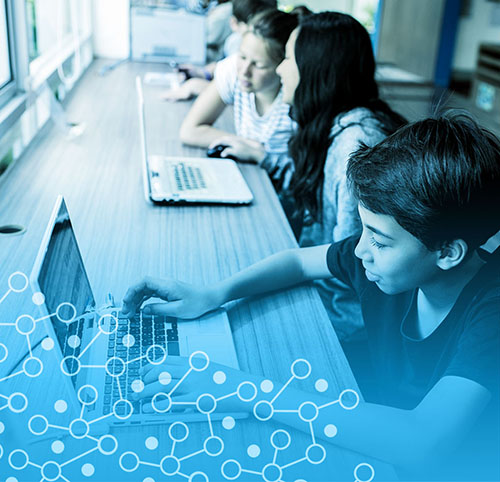
(1)* Heissel, J. (2016). The relative benefits of live versus online delivery: Evidence from virtual algebra I in North Carolina. Economics of Education Review, 53, 99-115. doi:10.1016/j.econedurev.2016.05.001
(2)* Vanlehn, K. (2011). The Relative Effectiveness of Human Tutoring, Intelligent Tutoring Systems, and Other Tutoring Systems. Educational Psychologist, 46(4), 197-221. doi:10.1080/00461520.2011.611369
(3)* OECD. (2015). Students, computers and learning; Making the connection. Retrieved from: http://www.oecd.org/publications/students-computers-and-learning-9789264239555-en.htm.
(4)* Rowan, C. (2010). Unplug – don’t drug: A critical look at the influence of technology on child behavior with an alternative way of responding other than evaluation and drugging. Ethical Human Psychology and Psychiatry, 12(1). Pp. 60-68. https://doi.org/10.1891/1559-4343.12.1.60. Walsh, J. (2018). Associations between 24 hour movement behaviours and global cognition in US children: A cross-sectional observational study. The Lancet Child & Adolescent Health, 2(11). https://doi.org/10.1016/S2352-4642(18)30278-5
(5)* Alghamdi, Y. (2016). The negative effects of technology on children. Oakland University. Retrieved from: https://www.researchgate.net/publication/318851694_Negative_Effects_of_Technology_on_Children_of_Today. Zimmerman, F. et al. (2007). Associations Between Media Viewing and Language Development in Children Under Age 2 Years. The Journal of Pediatrics, 151(4) , 364-368. https://doi.org/10.1016/j.jpeds.2007.04.071
(6)* King, P. & R. Behnke, R. (1999). Technology-based instructional feedback intervention. Educational Technology, 39. Mason, B.P., & Bruning, R. (2003). Providing feedback in computer-based instruction: What research tells us. Swart, R. (2017). Purposeful use of technology to support critical thinking. JOJ Nurse Health Care, 4(1). https://juniperpublishers.com/jojnhc/pdf/JOJNHC.MS.ID.555626.pdf
(7)* Konnikova, M. (2012, April 30). On writing, memory, and forgetting: Socrates and Hemingway take on Zeigarnik [Web log post]. Retrieved January 12, 2019, from: https://blogs.scientificamerican.com/literally-psyched/on-writing-memory-and-forgetting-socrates-and-hemingway-take-on-zeigarnik/
(8)* Gershon, L. (2017, December 28). How blackboards transformed American education. JSTOR Daily. Retrieved January 12, 2019, from: https://daily.jstor.org/how-blackboards-transformed-american-education/
(9)* GAhn, S. J., Bostick, J., Ogle, E., Nowak, K. L., Mcgillicuddy, K. T., & Bailenson, J. N. (2016). Experiencing Nature: Embodying Animals in Immersive Virtual Environments Increases Inclusion of Nature in Self and Involvement With Nature. Journal of Computer-Mediated Communication, 21 (6), 399-419. doi:10.1111/jcc4.12173education/
(10)* Desmarais, M. C., & Baker, R. S. (2011). A review of recent advances in learner and skill modeling in intelligent learning environments. User Modeling and User-Adapted Interaction, 22 (1-2), 9-38. doi:10.1007/s11257-011-9106-8
(11)* Hossain, Z., Bumbacher, E., Blikstein, P., & Riedel-Kruse, I. (2017). Authentic science inquiry learning at scale enabled by an Interactive biology cloud experimentation lab. Proceedings of the Fourth (2017) ACM Conference on Learning @ Scale – L@S 17. doi:10.1145/3051457.3053994
(12)* Jabr, F. (2013, April 11). The reading brain in the bigital age: The science of paper versus screens [Web log post]. Retrieved January 12, 2019, from: https://www.scientificamerican.com/article/reading-paper-screens/
(13)* Mangen, A., Walgermo, B. R., & Brønnick, K. (2013). Reading linear texts on paper versus computer screen: Effects on reading comprehension. International Journal of Educational Research, 58, 61-68. doi:10.1016/j.ijer.2012.12.002
(14)* Ackerman, R., & Lauterman, T. (2012). Taking reading comprehension exams on screen or on paper? A metacognitive analysis of learning texts under time pressure. Computers in Human Behavior, 28 (5), 1816-1828. doi:10.1016/j.chb.2012.04.02
Lauterman, T., & Ackerman, R. (2014). Overcoming screen inferiority in learning and calibration. Computers in Human Behavior, 35, 455-463. doi:10.1016/j.chb.2014.02.0463
(15)* Ragan, E. D., Jennings, S. R., Massey, J. D., & Doolittle, P. E. (2014). Unregulated use of laptops over time in large lecture classes. Computers & Education, 78, 78-86. doi:10.1016/j.compedu.2014.05.002
(16)* Sana, F., Weston, T., & Cepeda, N. J. (2013). Laptop multitasking hinders classroom learning for both users and nearby peers. Computers & Education, 62, 24-31. doi:10.1016/j.compedu.2012.10.003
(17)* Sana, F., Weston, T., & Cepeda, N. J. (2013). Laptop multitasking hinders classroom learning for both users and nearby peers. Computers & Education, 62, 24-31. doi:10.1016/j.compedu.2012.10.003
(18)* Sana, F., Weston, T., & Cepeda, N. J. (2013). Laptop multitasking hinders classroom learning for both users and nearby peers. Computers & Education, 62, 24-31. doi:10.1016/j.compedu.2012.10.003
(19)* Herodotou, C. (2017). Young children and tablets: A systematic review of effects on learning and development. Journal of Computer Assisted Learning, 34 (1), 1-9. doi:10.1111/jcal.12220
(20)* Li, Q., & Ma, X. (2010). A Meta-analysis of the Effects of Computer Technology on School Students’ Mathematics Learning. Educational Psychology Review, 22 (3), 215-243. doi:10.1007/s10648-010-9125-8
(21)* Berkowitz, T., Schaeffer, M. W., Maloney, E. A., Peterson, L., Gregor, C., Levine, S. C., & Beilock, S. L. (2015). Math at home adds up to achievement in school. Science, 350 (6257), 196-198. doi:10.1126/science.aac7427
(22)* Ma, W., Adesope, O. O., Nesbit, J. C., & Liu, Q. (2014). Intelligent tutoring systems and learning outcomes: A meta-analysis. Journal of Educational Psychology, 106 (4), 901-918.
http://dx.doi.org/10.1037/a0037123
(23)* Grinager, H. (2006). How education technology leads to improved student achievement (USA). Denver, CO: Education Technology Partners: Technology in K-12 Education. Retrieved January 12, 2019, from: http://www.ncsl.org/
(24)* Carr, C. S. (2003). Visualizing argumentation: Software tools for collaborative and educational sense-making (P. A. Kirschner, S. J. Buckingham Shum, & P. A. Kirschner, Eds.). Retrieved January 12, 2019, from: https://www.springer.com/gp/book/9781852336646
(25)* Blikstein, P., & Wilensky, U. (2007). Bifocal modeling: A framework for combining computer modeling, robotics and real-world sensing. Retrieved January 12, 2019, from: https://ccl.northwestern.edu//2007/09-bifocal_modeling.pdf. Hernández-Ramos, P., & Paz, S. D. (2009). Learning history in middle school by designing multimedia in a project-based learning experience. Journal of Research on Technology in Education,42 (2), 151-173. doi:10.1080/15391523.2009.10782545
(26)* Kuiper, E., & Pater-Sneep, M. D. (2014). Student perceptions of drill-and-practice mathematics software in primary education. Mathematics Education Research Journal, 26 (2), 215-236. doi:10.1007/s13394-013-0088-1
(27)* Wenglinsky, H. (1998). Does it compute? The relationship between educational technology and student achievement in mathematics. Retrieved from: https://www.ets.org/Media/Research/pdf/PICTECHNOLOG.pdf
Also Wenglinsky, H. (2005). Using technology wisely: The keys to success in schools. New York: Teachers College.
(28)* Wenglinsky, H. (2005). Technology and achievement: the bottom line. Learning in the digital age, 63 (4), 29-32. Retrieved January 12, 2019, from: https://imoberg.com.
(29)* Zhang, T., Xie, Q., Park, B., Kim, Y., Broer, M., & Bohrnstedt, G. (2016). Computer familiarity and its relationship to performance in three NAEP digital-based assessments (Working paper No. 01-2016). Washington, DC: American Institutes for Research (AIR). Retrieved January 12, 2019, from: https://www.air.org
(30)* Programme for International Student Assessment (PISA). (2018). Retrieved January 12, 2019, from: http://www.oecd.org/pisa/aboutpisa/
(31)* NAEP, National Assessment of Educational Progress (2017). Retrieved from: http://nces.ed.gov/nationsreportcard/
(32)* Pike, G. R. (1995). The relationship between self reports of college experiences and achievement test scores. Research in Higher Education, 36 (1), 1-21. doi:10.1007/bf02207764
(33)* Yienger, M. E. (2016). Too much tech harms reading retention in young children. Inquiries journal/student pulse, 8(03). Retrieved from: http://www.inquiriesjournal.com/a?id=1374
(34)* Hull, M., & Duch, K. (2019). One-to-one technology and student outcomes: evidence from Mooresville’s digital conversion initiative. Educational Evaluation and Policy Analysis, 41 (1), 79–97. https://doi.org/10.3102/0162373718799969. OECD (2015). Students, Computers, and Learning: Making the Connection, PISA, OECD Publishing. Retrieved from: https://read.oecd-ilibrary.org/education/students-computers-and-learning_9789264239555-en#page4. Ravizza, S. M., Uitvlugt, M. G., & Fenn, K. M. (2017). Logged in and zoned Out: How laptop Internet use relates to classroom learning. Psychological Science, 28 (2), 171–180. https://doi.org/10.1177/0956797616677314
(35)* Zheng, B., Warschauer, M., Lin, C.-H., & Chang, C. (2016). Learning in One-to-one laptop environments: A meta-analysis and research synthesis. Review of Educational Research, 86 (4), 1052–1084. https://doi.org/10.3102/0034654316628645.
Tamim, R. M., Bernard, R. M., Borokhovski, E., Abrami, P. C., & Schmid, R. F. (2011). What forty years of research says about the Impact of technology on learning: A second-order meta-analysis and validation study. Review of Educational Research, 81(1), 4–28. https://doi.org/10.3102/0034654310393361.
(36)* Hesse, L. (2017). The effects of blended learning on K-12th grade students. Graduate Research Papers. Retrieved from: https://scholarworks.uni.edu/cgi/viewcontent.cgi?article=1116&context=grp. Utami, I. (2018). The effect of blended learning model on senior high school students’ achievement. Universitas Bung Hatta, Retrieved from: https://www.shs-conferences.org/articles/shsconf/pdf/2018/03/shsconf_gctale2018_00027.pdf
(37)* Carter, S. et al. (2016). The impact of computer usage on academic performance: Evidence from a randomized trial at the United States Military Academy. National Bureau of Economic research. Retrieved from: https://seii.mit.edu/wp-content/uploads/2016/05/SEII-Discussion-Paper-2016.02-Payne-Carter-Greenberg-and-Walker-2.pdf. Sana, F. et al. (2013). Laptop multitasking hinders classroom learning for both users and nearby peers. Computers and Education, 62. Pp. 24-31. https://www.sciencedirect.com/science/article/pii/S0360131512002254?via%3Dihub
(38)* Walsh, J. (2018). Associations between 24 hour movement behaviours and global cognition in US children: A cross-sectional observational study. The Lancet Child & Adolescent Health, 2 (11). https://doi.org/10.1016/S2352-4642(18)30278-5
(39)* Escueta, M. et al. (2017). Education technology: An evidence-based review. National Bureau of Economic research. Retrieved from: https://www.nber.org/papers/w23744.pdf. Tomasik, M. J., Berger, S., & Moser, U. (2018). On the development of a computer-based tool for formative student assessment: epistemological, methodological, and practical issues. Frontiers in psychology, 9, 2245. doi:10.3389/fpsyg.2018.02245. Shute, V.J. & Rahimi, S. (2017). Journal of Computer Assisted Learning. 33. Retrieved from: http://myweb.fsu.edu/vshute/pdf/jcal.pdf. Reimann, P. et al. (2011). Design of a computer-assisted assessment system for classroom formative assessment. Retrieved from: http://citeseerx.ist.psu.edu/viewdoc/download?doi=10.1.1.228.2632&rep=rep1&type=pdf
(40)* International Journal of Education and Development using Information and Communication Technology. (IJEDICT), 2010, Vol. 6, Issue 1, pp. 76-87. Retrieved from: https://files.eric.ed.gov/fulltext/EJ1084978.pdf
(41)* Swart, R. (2017). Purposeful use of technology to support critical thinking. JOJ Nurse Health Care, 4 (1). https://juniperpublishers.com/jojnhc/pdf/JOJNHC.MS.ID.555626.pdf
(42)* Dixon, F., Cassady, J., Cross, T., & Williams, D. (2005). Effects of technology on critical thinking and essay writing among gifted adolescents. Journal of Secondary Gifted Education, 16 (4), 180–189. https://doi.org/10.4219/jsge-2005-482. O’Dwyer, L. M., Russell, M., Bebell, D., & Tucker-Seeley, K. R. (2005). Examining the relationship between home and school computer use and students’ English/language arts test scores. Journal of Technology, Learning, and Assessment, 3 (3). Retrieved from: https://pdfs.semanticscholar.org/c849/3a5ac5d569a7f41366a58e4f5b3a73790668.pdf. Wenglinsky, H. (1998). Does it compute? The relationship between educational technology and student achievement in mathematics. Educational Testing Service. Retrieved from: https://files.eric.ed.gov/fulltext/ED425191.pdf
(43)* Carbonneau, K. J., Marley, S. C., & Selig, J. P. (2013). A meta-analysis of the efficacy of teaching mathematics with concrete manipulatives. Journal of Educational Psychology, 105 (2), 380-400. doi:10.1037/a0031084
(44)* Ma, W., Adesope, O. O., Nesbit, J. C., & Liu, Q. (2014). Intelligent tutoring systems and learning outcomes: A meta-analysis. Journal of Educational Psychology, 106 (4), 901-918. doi:10.1037/a0037123
Steenbergen-Hu, S., & Cooper, H. (2014). A meta-analysis of the effectiveness of intelligent tutoring systems on college students’ academic learning. Journal of Educational Psychology, 106 (2), 331-347. doi:10.1037/a0034752
(45)* Santiago, H. (2011). Visual mapping to enhance learning and critical thinking skills. Optometric Education, 36 (3). Retrieved from: https://journal.opted.org/articles/Volume_36_Number_3_VisualMapping.pdf
(46)* Faste, H., & Lin, H. (2012). The untapped promise of digital mind maps. CHI.
(47)* Stanhope, D., & Rectanus, K. (2016). Educational technology: What 49 schools discovered about usage when the data were uncovered. EDM.
(48)* Carr, C. S. (2003). Visualizing argumentation: Software tools for collaborative and educational sense-making (P. A. Kirschner, S.J. Buckingham-Shum, & C.S Carr, Eds.). Retrieved January 12, 2019, from: https://www.springer.com/gp/book/9781852336646
(49)* Magnisalis, I., Demetriadis, S., & Karakostas, A. (2011). Adaptive and intelligent systems for collaborative learning support: A review of the field. IEEE Transactions on Learning Technologies, 4(1), 5-20. doi:10.1109/tlt.2011.2